Healthcare Datasets for Machine Learning: A Game Changer in Business Solutions
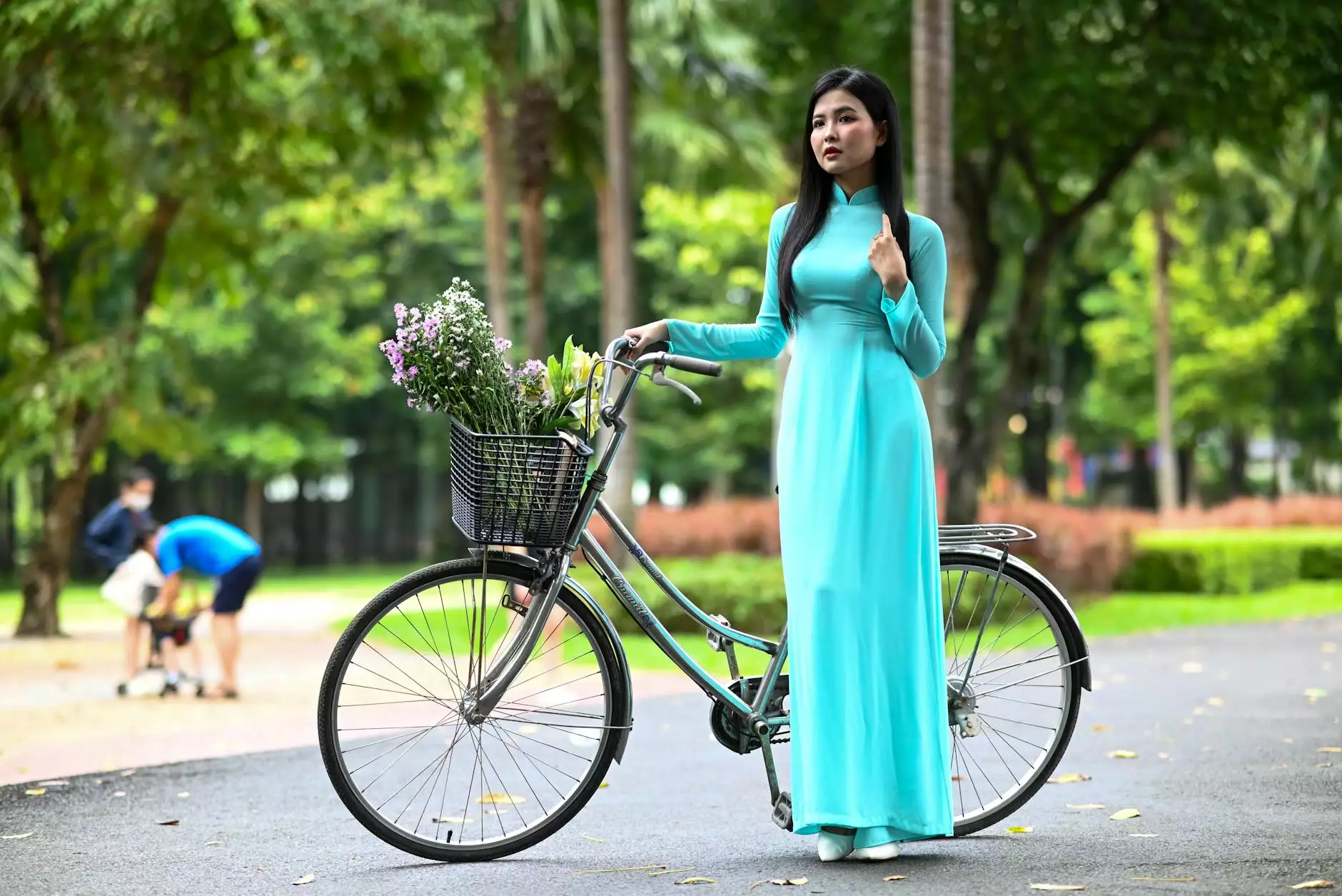
In today's data-driven world, healthcare datasets for machine learning are becoming increasingly essential for businesses looking to enhance their service offerings. The integration of machine learning in various sectors, including home services and locksmiths, can vastly improve operational efficiency and customer satisfaction. This article explores the multifaceted benefits of leveraging healthcare datasets for machine learning and how they can transform business practices, particularly on platforms like keymakr.com.
Understanding Machine Learning and Its Relevance to Healthcare Data
Machine learning (ML) involves using algorithms that allow computers to learn from data and make predictions based on that data. In the realm of healthcare datasets, this technology can analyze large volumes of information to uncover patterns and insights that might otherwise go unnoticed.
Types of Healthcare Datasets for Machine Learning
Healthcare datasets vary widely in their structure and content. Below are some common types:
- Electronic Health Records (EHRs): Comprehensive patient records containing treatment histories, medication details, and diagnostic data.
- Medical Imaging Datasets: Collections of medical images used for diagnosing diseases, such as X-rays, MRIs, and CT scans.
- Genomic Data: Information about individual genetic makeups that can help personalize treatment plans.
- Clinical Trial Data: Results and insights from clinical studies that are crucial for understanding treatment outcomes.
The Importance of Healthcare Datasets for Machine Learning in Business
The inclusion of healthcare datasets for machine learning in business strategies, especially within home services, locksmiths, and related fields, offers transformative benefits.
Improved Customer Insights
One of the primary advantages of utilizing healthcare datasets is the ability to gain deep insights into customer needs and behaviors. For example, a locksmith business can analyze data related to past customer interactions, understanding which services are most in demand and predicting future trends. This proactive approach allows businesses to tailor their services to align with customer expectations.
Enhanced Decision-Making
Data-backed decision-making leads to more strategic investments. By leveraging healthcare datasets, businesses can evaluate the effectiveness of their services and identify areas needing improvement. This analytical approach minimizes risks and resource wastage, allowing companies to focus on scalable strategies.
Practical Applications of Healthcare Datasets in Home Services
Integrating healthcare datasets for machine learning into home services can take various forms, each adding unique value:
Predictive Analytics for Service Demand
Using machine learning models to analyze historical data can help predict future service demands. For example, by examining seasonal trends and local health crises, locksmiths and home service providers can anticipate spikes in demand. This foresight enables businesses to manage staffing and inventory more effectively.
Optimizing Operational Efficiency
Deploying machine learning algorithms can optimize route planning for service delivery, reducing travel time and costs. By analyzing past data on service appointments and geographical distribution, businesses can determine the most efficient routes and schedules.
Improving Customer Support
Data-driven insights can empower customer support teams to provide better service. By analyzing customer feedback collected through various channels, businesses can quickly address the most common issues and enhance their overall service quality.
Challenges of Implementing Machine Learning with Healthcare Datasets
While the prospects of using healthcare datasets for machine learning in business are promising, companies must also navigate several challenges:
Data Privacy and Security
Handling sensitive healthcare data necessitates strict adherence to privacy regulations. Businesses must ensure robust security measures to protect customer information and maintain trust while using these datasets.
Data Quality and Standardization
The effectiveness of machine learning hinges on the quality of the data being analyzed. Ensuring that datasets are accurate, complete, and properly formatted is crucial. Businesses often face challenges in standardizing data from different sources.
Integration with Existing Systems
Integrating machine learning technologies with existing business processes can be complex. Organizations must be ready to invest in training and technology to ensure a smooth transition.
Strategies for Effective Use of Healthcare Datasets
To fully harness the potential of healthcare datasets for machine learning, businesses should consider the following strategies:
- Invest in Data Analytics Tools: Equip your team with tools that can seamlessly analyze healthcare datasets and provide actionable insights.
- Collaborate with Data Scientists: By working with experts in data science, companies can better understand how to implement machine learning effectively.
- Focus on Continuous Learning: The field of machine learning is constantly evolving. Ongoing training and development for staff are crucial in keeping pace with advancements.
- Prioritize Data Governance: Establish data governance practices to ensure compliance and ethical use of data.
Case Studies: Successful Implementation of Machine Learning Using Healthcare Datasets
Numerous businesses have successfully leveraged healthcare datasets for machine learning, yielding impressive results. Here are a few case studies highlighting their impact:
Case Study 1: Predicting Service Needs in Locksmiths
A regional locksmith company utilized historical data on customer requests to build predictive models that forecasted service needs based on time of year and local events. This proactive strategy allowed them to enhance their workforce planning, ensuring they had the right number of technicians available during peak times, leading to a 30% increase in response efficiency.
Case Study 2: Enhancing Customer Engagement in Home Services
A home cleaning service compiled customer satisfaction surveys and integrated machine learning algorithms to analyze feedback trends. By identifying common service complaints, they revamped their training programs, which improved their customer satisfaction ratings by over 25% in just six months.
Conclusion: The Future of Business with Healthcare Datasets for Machine Learning
As the landscape of business continues to evolve, the importance of utilizing healthcare datasets for machine learning cannot be understated. From predictive analytics to optimizing operational practices, the potential for improved service delivery is immense. Businesses like Keymakr stand to benefit greatly from these advancements, positioning themselves at the forefront of innovation in their respective fields.
With the right approach to data management, privacy considerations in place, and a commitment to harnessing the full potential of machine learning, businesses can not only survive but thrive in this new data-centric era. The road ahead is promising, and those who embrace the change are sure to reap the rewards.